Use Case - Machine Learning
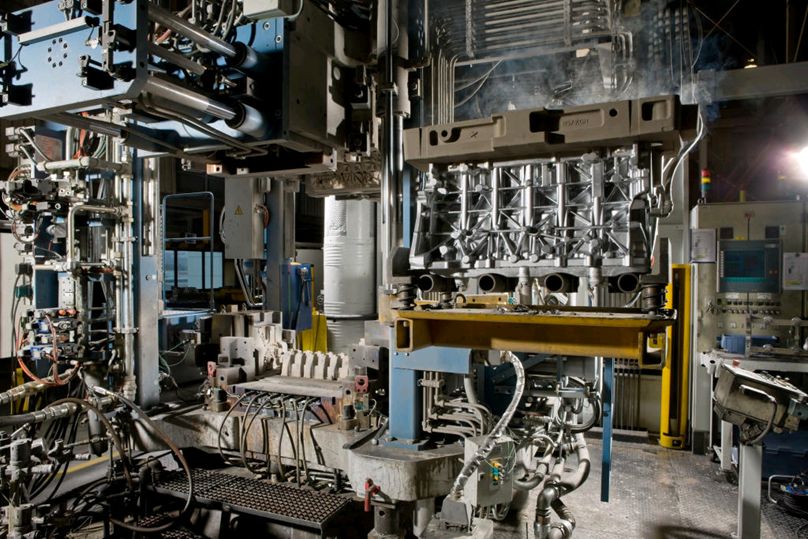
AI environment from which SMEs also benefit
German SMEs are still lagging behind in the implementation of AI strategies. This is the conclusion of a recent IT study by Computerwoche.[1] While many large companies are already using the advantages of the smart factory, medium-sized industrial companies are still hesitant.
The vision of AI has found its way into German industrial production. In particular, the core industrial sectors – i.e. the automotive and mechanical engineering industries as well as the chemical and electrical industries – have immense interest in the possibilities and opportunities offered by AI. According to the digital association Bitkom, four out of ten companies in Germany use AI applications.[2]
John Deere on the way to the smart factory
Since the beginning of 2016, the tractor manufacturer John Deere has been gradually converting its plant in Mannheim into a self-learning factory. The knowledge gained from the Internet of Things will then also be transferred to other group locations. Together with the project partner IBM, new modules are developed every six weeks using agile methods. Lower costs and more flexible processes are to be the main improvements, as well as a lower reject rate. In Mannheim, parts are therefore identified using RFID and NFC, and in some cases the components are checked by cameras. The tractor manufacturer uses smart glasses to ensure that no incorrect or faulty components are selected in the warehouse. This has reduced the error rate in the warehouse to zero. In the future, the smart factory will enhance the area of business intelligence thanks to IBM’s Watson technology. Employees can then ask Watson specific questions directly by voice input, to which the computer will hopefully have the right solution ready.
Raising awareness of AI among SMEs
Although SMEs do not deny the advantages of AI applications and strategies, decision-makers are finding it difficult to implement them. According to the digital association Bitkom, the biggest hurdle in the use of AI is the funds required for this.[3] Three quarters of all industrial companies say that the high investment costs inhibit the use of AI in the company. Data protection and data security requirements are also among the main obstacles, at 55 and 51 percent respectively. The lack of skilled workers is also a problem (53 percent).
Most large companies are currently integrating mainly stand-alone solutions for cost reasons. According to Computerwoche, the most popular topics are an increase in efficiency through the acceleration of existing processes, better maintenance (predictive maintenance) and the networking of all process chains. In this way, acute problems can be solved and significant company successes can already be achieved.
BMW, for example, has reduced scrap for a light metal foundry and at the same time reduced the necessary but expensive testing processes. In order to better understand the actual production process and eliminate the problems airsing from this, the company now working with the IBM SPSS Modeler as part of watsonx.ai.
Optimising production with machine learning
Machine learning is used to analyse large quantities of input parameters. This makes it possible to reduce quality inspection costs, for example. At the same time, however, it can be used to increase the overall quality of production. The analysis methods reduce the reject rate enormously. The large data accumulating in the production process are now analysed in such a way that they provide insights into the influencing factors of the individual production steps as well as insights into the quality of the end products. The new insights enable process adjustments to be initiated early and in a targeted manner. Today, data results that previously took days are available in just a few hours. For example, tools have to be replaced less often, which saves time and money. Given the high stability of the manufacturing process, the 24-hour evaluation cycle is tantamount to real-time monitoring. BMW was able to reduce the scrap rate by 80 per cent within 12 weeks.AI
BMW Case Study
In our BMW Case Study you can read how the BMW Group uses the IBM® SPSS® Modeler machine learning environment to analyse and evaluate daily data and thus optimise its production. You are welcome to request this case study free of charge via our contact form.
No project has failed so far
Experts expect an increase in AI projects, also in SMEs, within the next few years. The successful projects so far give reason for optimism. Not a single project has failed. Almost a quarter of the companies were able to record positive effects immediately after project completion. Most of the projects achieved their goals within a year.
For more information on the topic of IBM Analytics, including for warehouse and production optimisation, visit the Business Analytics Forum on 14 June 2017 at the Hotel Stadt Hameln.
[1] Computerwoche: Studie: „Industrie 4.0 2017 – Wo steht Deutschland?“, https://www.computerwoche.de/a/industrie-4-0-grosse-unternehmen-sind-pioniere-mittelstand-muss-aufholen,3330657, 2017
[2] Bitkom-RESEARCH, „4 von 10 Unternehmen nutzen Industrie 4.0-Anwendungen“, http://www.bitkom-research.de/Presse/Pressearchiv-2015/4-von-10-Unternehmen-nutzen-Industrie-40-Anwendungen
[3] https://www.bitkom.org/Presse/Presseinformation/Fast-jedes-zweite-Industrieunternehmen-nutzt-Industrie-40.html